Method
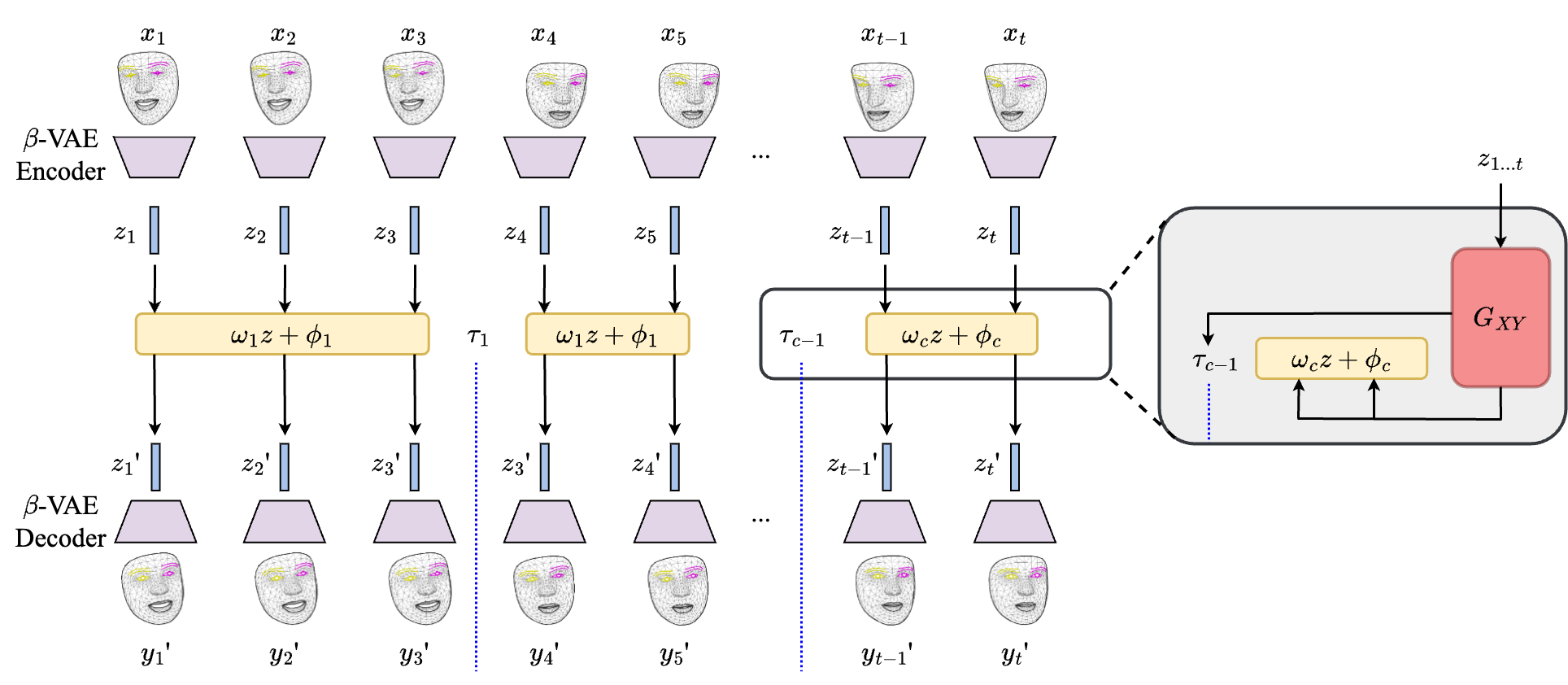
Do our facial expressions change when we speak over video calls? Given two unpaired sets of videos of people, we seek to automatically find spatio-temporal patterns that are distinctive of each set. Existing methods use discriminative approaches and perform post-hoc explainability analysis. Such methods are insufficient as they are unable to provide insights beyond obvious dataset biases, and the explanations are useful only if humans themselves are good at the task. Instead, we tackle the problem through the lens of generative domain translation: our method generates a detailed report of learned, input-dependent spatio-temporal features and the extent to which they vary between the domains. We demonstrate that our method, FacET (Facial Explanations through Translations), can discover behavioral differences between conversing face-to-face (F2F) and on video-calls (VCs). We also show the applicability of our method on discovering differences in presidential communication styles. Additionally, we are able to predict temporal change-points in videos that decouple expressions in an unsupervised way, and increase the interpretability and usefulness of our model. Finally, our method, being generative, can be used to transform a video call to appear as if it were recorded in a F2F setting. Experiments and visualizations show our approach is able to discover a range of behaviors, taking a step towards deeper understanding of human behaviors.
@misc{sarin2024videomeetingschangeexpression,
title={How Video Meetings Change Your Expression},
author={Sumit Sarin and Utkarsh Mall and Purva Tendulkar and Carl Vondrick},
year={2024},
eprint={2406.00955},
archivePrefix={arXiv},
primaryClass={cs.CV},
url={https://arxiv.org/abs/2406.00955},
}